企業數據不斷變化。隨著時間的推移,這給保持 AI 系統的準確性帶來了重大挑戰。隨著企業組織越來越依賴 代理式 AI 系統 來優化業務流程,保持這些系統與不斷變化的業務需求和新數據保持一致變得至關重要。
本文將深入探討如何使用 NVIDIA NeMo 微服務構建數據飛輪迭代,并簡要概述構建端到端流程的步驟。如需了解如何使用 NeMo 微服務解決構建數據飛輪時面臨的各種挑戰,請參閱使用 NVIDIA NeMo 微服務更大限度地提高 AI Agent 性能。
為什么 data flywheels 對于代理式 AI 至關重要
數據飛輪是一種自我增強的循環。從用戶交互中收集的數據可改進 AI 模型,從而提供更好的結果,吸引更多用戶生成更多數據,從而在持續改進循環中進一步增強系統。這類似于獲取經驗和收集反饋以學習和改進工作的過程。
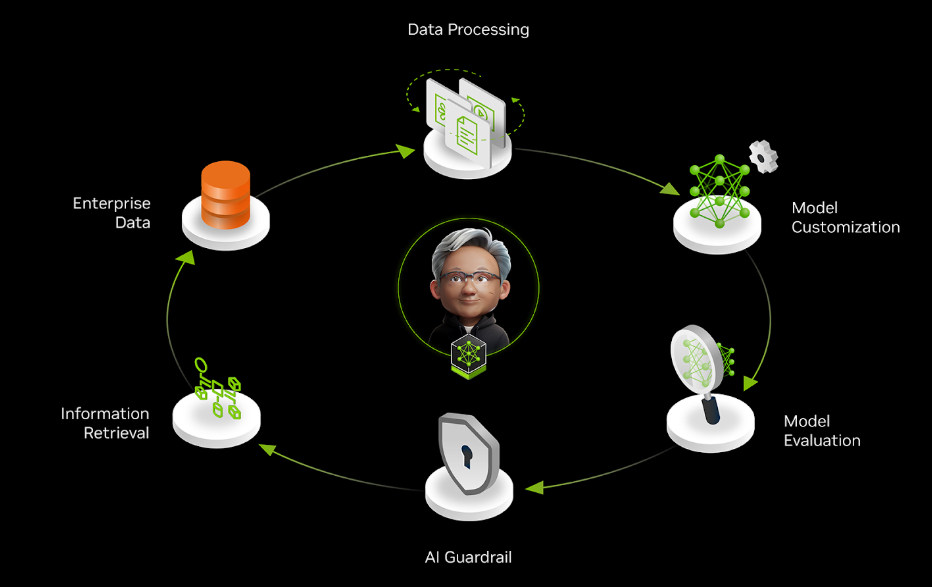
因此,需要部署的應用持續適應并保持高效是將數據 flywheel 整合到代理式系統中的主要動機。
需要持續適應
在生產環境中,AI 應用面臨著持久的挑戰:model drift。設想一個將用戶查詢路由到專業專家系統的 AI 智能體 。此系統的輸入、所使用的工具及其響應都在不斷演變。如果沒有適應機制,準確度必然會下降,原因如下:
- 更新企業知識庫和文檔
- 改變用戶行為和查詢模式
- 更改工具 API 和響應
例如,當組織添加具有不同模式的新 MongoDB 數據集并更新其響應格式時,通過查詢事務 SQL (PostgreSQL) 數據庫來回答客戶問題的 銀行大語言模型 (LLM) 智能體 將面臨重大挑戰。在不進行重新訓練的情況下,智能體繼續為舊數據庫結構制定查詢,從而導致檢索失敗或信息錯誤。這會損害客戶信任,并可能造成合規性問題。
效率需求
隨著這些智能體處理更復雜的任務變得越來越復雜,保持準確性和相關性變得更具挑戰性。此外,隨著交易量的增加,為這些模型提供服務的計算成本也會大幅增加,因此效率問題至關重要。這對于代理式 AI 系統來說尤其成問題,因為它們通常需要多個推理通道來執行推理、規劃和執行步驟,與簡單的單通道推理模型相比,計算負擔成倍增加。
當智能體必須評估多個潛在動作、查詢多個知識來源并驗證其輸出時,每次交互所需的計算量可能比標準模型推理多 5x 到 10x 倍,隨著使用規模的擴大,基礎設施成本會大幅增加。
使用定制技術,您可以優化較小的模型,使其與較大模型的準確性相匹配,從而降低延遲和總體擁有成本 (TCO) 。此外,隨著更新和功能更強大的模型的出現,不斷評估這些模型 (及其微調的變體) ,利用用戶交互數據可以確保持續的性能和適應性。
使用 NVIDIA NeMo 微服務為您的數據飛輪提供支持
NVIDIA NeMo 微服務提供了一個用于構建數據飛輪的端到端平臺,使企業能夠利用最新信息不斷優化其 AI 智能體。
如圖 2 所示, NVIDIA NeMo 可幫助企業 AI 開發者輕松地大規模整理數據,使用熱門的微調技術自定義 LLM,根據行業和自定義基準一致評估模型,并保護它們以獲得適當的接地輸出。
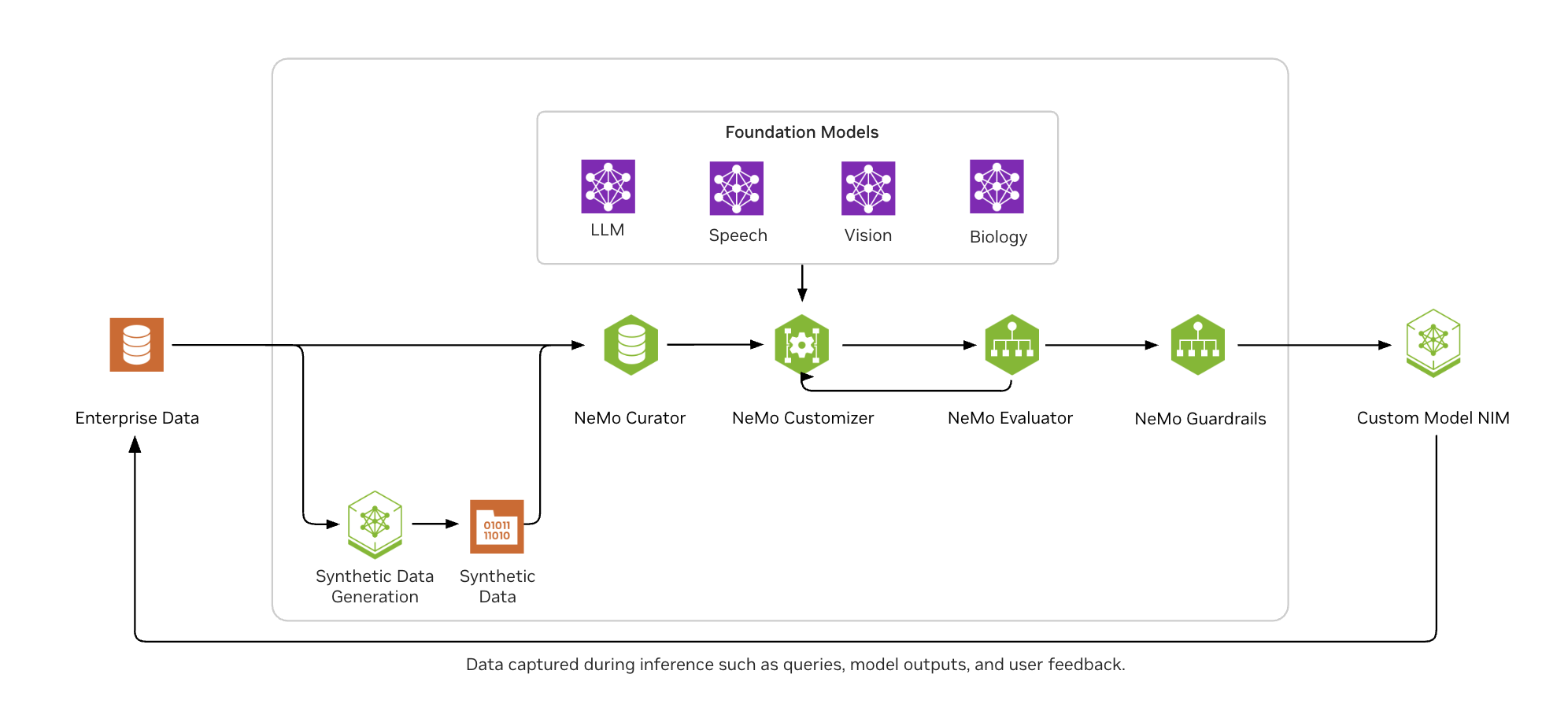
使用 NeMo 微服務增強 Agent 工具調用的示例代碼
為了說明 NeMo 微服務的端到端工作流,以智能體中的工具調用為例。工具調用使 LLM 能夠與外部系統交互、執行程序,以及訪問訓練數據中不可用的實時信息。
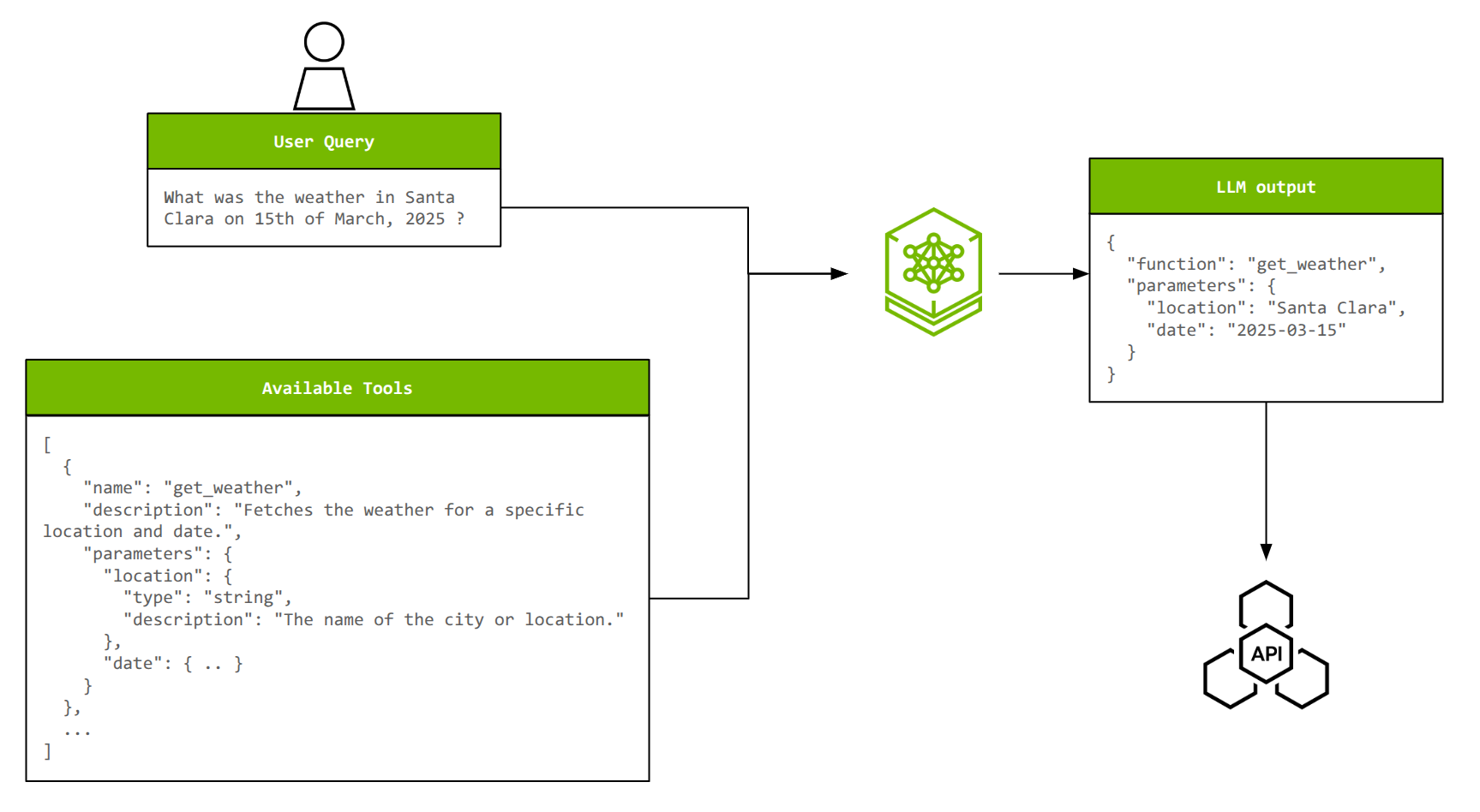
為了有效地調用工具,LLM 必須從可用選項中選擇正確的工具,從自然語言查詢中提取適當的參數,并且可能會將多個操作關聯在一起或并行調用多個工具。隨著工具數量及其復雜性的增加,定制對于保持準確性和效率至關重要。
通過在 xLAM 數據集 (~60,000 工具調用示例) 上微調 Llama 3.2 1B Instruct 模型 ,可以實現接近 Llama 3.1 70B Instruct 模型 的工具調用準確性,從而將模型大小減少 70x。
以下各節將概述關鍵步驟,以便您快速了解相關內容。查看 Jupyter notebooks 中的完整教程。
第 1 步:部署 NVIDIA NeMo 微服務
NeMo 微服務平臺以 Helm 圖表的形式提供,您可以選擇在支持 Kubernetes 的系統上進行部署。 首先 ,您可以在配備至少兩個 NVIDIA GPU (NVIDIA A100 80 GB 或 NVIDIA H100 80 GB) 的單節點 NVIDIA GPU 集群上使用 minikube。
第 2 步:數據準備
xLAM 數據集轉換為與用于訓練的 NeMo Customizer 和用于測試的 NeMo Evaluator 兼容的格式。每個數據樣本都是一個 JSON 對象,由用戶查詢、可用工具列表 (及其描述和參數) 和真值響應 (帶參數的選定工具) 組成。此外,還創建了用于訓練、驗證和測試的數據拆分。
NeMo Customizer 的數據格式如下所示。請注意,messages
包含用戶查詢和助手的 ground truth 響應,而 tools
包含可供選擇的可用工具列表。
{ "messages": [ { "role": "user", "content": "Where can I find live giveaways for beta access?" }, { "role": "assistant", "tool_calls": [ { "id": "call_beta", "type": "function", "function": { "name": "live_giveaways_by_type", "arguments": {"type": "beta"} } }, ] } ], "tools": [ { "type": "function", "function": { "name": "live_giveaways_by_type", "description": "Retrieve live giveaways from the GamerPower API based on the specified type.", "parameters": { "type": "object", "properties": { "type": { "type": "string", "description": "The type of giveaways to retrieve (e.g., game, loot, beta).", "default": "game" } }, "required": [] } } } ] } |
NeMo Evaluator 非常嚴格地遵循這種格式,但略有不同。有關更多信息,請參閱 Jupyter notebook 。
第 3 步:實體管理
NVIDIA NeMo 實體商店微服務可管理命名空間、項目、數據集和模型等組織實體,為高效資源管理提供分層結構。這樣一來,它可以實現無縫協作,并防止多個用戶之間的資源沖突。另一方面,NVIDIA NeMo 數據存儲微服務處理與這些實體關聯的實際文件,支持上傳、下載和版本控制等操作。
在此步驟中,準備好的數據集將通過與 Hugging Face Hub 接口 (HfApi) 支持的集成上傳到 NeMo Datastore,并通過 REST API 調用注冊到 Entity Store 和 Datastore。NeMo Customizer 和 Evaluator 引用這些路徑作為其輸入。
第 4 步:Low-rank adaptation (LoRA) 微調
NeMo Customizer 用于對 Llama 3.2 1B Instruct 模型進行 LoRA 微調。觸發自定義作業和監控作業狀態也是對 NeMo Customizer 端點的 REST API 調用。訓練參數可以像任何其他深度學習訓練作業一樣進行配置。此外,NeMo Customizer 與 Weights & Biases 無縫集成,用于監控訓練運行。
headers = { "wandb-api-key" : WANDB_API_KEY} if WANDB_API_KEY else None training_params = { "name" : "llama-3.2-1b-xlam-ft" , "output_model" : f "{NAMESPACE}/llama-3.1-8b-xlam-run1" , "config" : BASE_MODEL, "dataset" : { "name" : DATASET_NAME, "namespace" : NAMESPACE}, "hyperparameters" : { "training_type" : "sft" , "finetuning_type" : "lora" , "epochs" : 2 , "batch_size" : 16 , "learning_rate" : 0.0001 , "lora" : { "adapter_dim" : 32 , "adapter_dropout" : 0.1 } } } # Trigger the job. resp = requests.post(f "{NEMO_URL}/v1/customization/jobs" , json = training_params, headers = headers) customization = resp.json() # Used to track status JOB_ID = customization[ "id" ] # This will be the name of the model that will be used to send inference queries to CUSTOMIZED_MODEL = customization[ "output_model" ] |
第 5 步:推理
完成模型訓練后,其 LoRA 適配器將保存在 NeMo Entity Store 中,并由 NVIDIA NIM 自動獲取。您可以通過向模型的 NIM 端點發送提示來測試模型。
inference_client = OpenAI( base_url = f "{NIM_URL}/v1" , api_key = "None" ) completion = inference_client.chat.completions.create( model = CUSTOMIZED_MODEL, messages = test_sample[ "messages" ], tools = test_sample[ "tools" ], tool_choice = 'auto' , temperature = 0.1 , top_p = 0.7 , max_tokens = 512 , stream = False ) print (completion.choices[ 0 ].message.tool_calls) |
這將生成一個輸出,其中包含工具名稱以及填充其參數:
[ChatCompletionMessageToolCall( id = 'chatcmpl-tool-bd3e4ee65e0641b7ae2285a9f82c7aae' , function = Function(arguments = '{"type": "beta"}' , name = ’live_giveaways_by_type’), type = 'function' )] |
此時,模型已準備好進行評估,以量化其在 tool calling 時的準確性。
第 6 步:評估
使用 NeMo Evaluator 評估經過微調的模型,并將其準確性與基礎模型進行比較。function_name_accuracy
和 function_name_and_args_accuracy
等指標突出顯示了工具調用能力的改進。顧名思義,這些指標用于計算函數名稱及其參數的字符串匹配準確性。
評估通常包括以下幾個部分:
1. 創建評估配置:這將告知 NeMo Evaluator 有關所需評估的詳細信息,例如要使用的數據集、測試樣本數量、指標等。
simple_tool_calling_eval_config = { "type" : "custom" , "tasks" : { "custom-tool-calling" : { "type" : "chat-completion" , "dataset" : { "files_url" : f "hf://datasets/{NAMESPACE}/{DATASET_NAME}/testing/xlam-test.jsonl" , "limit" : 50 }, "params" : { "template" : { "messages" : "{{ item.messages | tojson}}" , "tools" : "{{ item.tools | tojson }}" , "tool_choice" : "auto" } }, "metrics" : { "tool-calling-accuracy" : { "type" : "tool-calling" , "params" : { "tool_calls_ground_truth" : "{{ item.tool_calls | tojson }}" } } } } } } |
2. 觸發評估作業: 這包括指定評估配置以及應評估的自定義模型 (NIM) 。
res = requests.post( f "{NEMO_URL}/v1/evaluation/jobs" , json = { "config" : simple_tool_calling_eval_config, "target" : { "type" : "model" , "model" : CUSTOM_MODEL_NAME} } ) base_eval_job_id = res.json()[ "id" ] |
3. 審查評估指標 :評估作業完成后,審查指標也是 REST 調用。
res = requests.get(f "{NEMO_URL}/v1/evaluation/jobs/{base_eval_job_id}/results" ) ft_function_name_accuracy_score = res.json()[ "tasks" ][ "custom-tool-calling" ][ "metrics" ][ "tool-calling-accuracy" ][ "scores" ][ "function_name_accuracy" ][ "value" ] ft_function_name_and_args_accuracy = res.json()[ "tasks" ][ "custom-tool-calling" ][ "metrics" ][ "tool-calling-accuracy" ][ "scores" ][ "function_name_and_args_accuracy" ][ "value" ] |
如果按照所提供的演示 Notebook 操作,您會發現與基本 meta/llama-3.2-1B-instruct
模型相比,出現了顯著的凸起。您可以大致預期:
function_name_accuracy
: 12% 至 92%function_name_and_args_accuracy
: 8% 至 72%
這非常接近此任務的基礎 meta/llama-3.1-70B-instruct
的準確性,一個模型是其大小的 70 倍。
第 7 步:護欄
此工作流的最后一部分是為您的自定義模型 NIM 添加護欄。在本例中,我們使用 nvidia/llama-3_1-nemoguard-8b-content-safety
來加強內容安全性,該方法可調節人類-LLM 交互內容,并將用戶提示和 LLM 響應分類為安全或不安全。如果內容不安全,模型還會返回一個響應,其中包含內容違反的類別列表。
與評估類似,這包括以下內容:
1. 創建和注冊 guardrails 配置: 請注意,系統會告知模型要檢測的不安全類別,以及模型應采用的響應格式。
data = { "name" : "toolcalling" , "namespace" : "default" , "data" : { "models" : [ { "type" : "content_safety" , "engine" : "nim" , "model" : "nvidia/llama-3.1-nemoguard-8b-content-safety" } ], "rails" : { "input" : { "flows" : [ "content safety check input $model=content_safety" ] }, "dialog" : { "single_call" : { "enabled" : False } } }, "prompts" : [ { "task" : "content_safety_check_input $model=content_safety" , "messages" : [ { "type" : "system" , "content" : "Task: Check if there is unsafe content in the user or agent messages in the below conversation in accordance with our safety policy with the below categories. Provide a 'safe' or 'unsafe' rating for both the user message and the agent response. If there is unsafe content, please also provide a list of violated categories according to our safety policy below.\n\n<BEGIN UNSAFE CONTENT CATEGORIES>\nS1: Violence.\nS2: Sexual.\nS3: Criminal Planning / Confessions.\nS4: Guns and Illegal Weapons.\nS5: Controlled / Regulated Substances.\nS6: Suicide and Self Harm.\nS7: Sexual (minor).\nS8: Hate / Identity Hate.\nS9: PII / Privacy.\nS10: Harassment.\nS11: Threat.\nS12: Profanity.\nS13: Needs Caution.\nS14: Other.\nS15: Manipulation.\nS16: Fraud / Deception.\nS17: Malware.\nS18: High Risk Gov Decision Making.\nS19: Political / Misinformation / Conspiracy.\nS20: Copyright / Trademark / Plagiarism.\nS21: Unauthorized Advice.\nS22: Illegal Activity.\nS23: Immoral / Unethical.\nS24: Deleting Logs.\n<END UNSAFE CONTENT CATEGORIES>\n\n<BEGIN CONVERSATION>\nuser: {{user_query}}\n<END CONVERSATION>\n\nProvide your safety assessment for the above conversation in the below output JSON format :\n{\ "User Safety\": safe or unsafe rating for the user message, \"Response Safety\" : safe or unsafe rating for the agent response. Omit if no agent response present. \ "Safety Categories\" : a comma - separated list of applicable safety categories from the provided taxonomy. Omit if all safe.}\n\nDo not include anything other than the output JSON in your response.\nOutput JSON:" }, { "type" : "user" , "content" : "{{ user_input }}" } ], "output_parser" : "nemoguard_parse_prompt_safety" , "max_tokens" : 50 } ] }, } # Register the guardrails configuration response = requests.post(GUARDRAILS_URL, json = data) |
2. 在 LLM NIM 推理調用之前為用戶輸入添加 guardrails
payload = { "model" : BASE_MODEL, "messages" : [ { "role" : "user" , "content" : user_message } ], "guardrails" : { "config_id" : "toolcalling" }, "temperature" : 0.2 , "top_p" : 1 } # Check for unsafe user message in guardrails response = requests.post(f "{NEMO_URL}/v1/guardrail/checks" , json = payload) status = response.json() if status = = “success”: # SAFE … (Proceed with your LLM inference call as in step 5 ) else : # UNSAFE print (f "Not a safe input, the guardrails have resulted in status as {status}. Tool-calling shall not happen" ) |
開始使用
按照本文中概述的步驟,您可以使用 NeMo 微服務為模型自定義、推理、評估和護欄構建端到端流程。如果此管道能夠自動處理定期觸發或在檢測到漂移時觸發的連續數據流,則需要建立數據飛輪。這種自增強循環使您的系統能夠不斷學習、適應和改進,從而不斷推動性能的持續提升。
NVIDIA NeMo 微服務現已正式開放下載。 下載微服務并使用 教程 notebook 和 相關視頻 ,開始使用本文中展示的示例。
如需詳細了解 NeMo 微服務,請 參閱文檔 。要在生產環境中運行 NeMo 微服務,請申請 NVIDIA AI Enterprise 的 90 天免費許可證 。
?