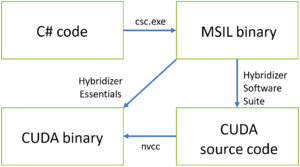
Hybridizer 是一個來自 Altimesh 的編譯器,它允許您從 C 代碼或. NET 程序集編程 GPUs 和其他加速器。使用修飾符號來表示并行性, Hybridizer 生成針對多核 CPUs 和 GPUs 優化的源代碼或二進制文件。在這篇博文中,我們展示了 CUDA 的目標。
圖 1 顯示了混合器編譯管道。使用 Parallel.For
等并行化模式,或者像在 CUDA 中那樣顯式地分配并行工作,您可以從加速器的計算馬力中獲益,而無需了解其內部架構的所有細節。
[EntryPoint] public static void Run(double[] a, double[] b, int N) { ? ?Parallel.For(0, N, i => { a[i] += b[i]; }); }
您可以使用 NVIDIA Nsight?Visual Studio Edition?在 GPU 上調試和分析這段代碼。Hybridizer 實現高級 C#功能,包括虛擬功能和泛型。
哪里可以獲取 Hybridizer
Hybridizer 有?兩個版本 :
- Hybridizer 軟件套件:啟用 CUDA 、 AVX 、 AVX2 、 AVX512 目標并輸出源代碼。可以查看此源代碼,這在投資銀行等一些業務中是強制性的。 Hybridizer 軟件套件是根據客戶 應要求 獲得許可的。
- 雜交者基本要素 :僅啟用 CUDA 目標并僅輸出二進制文件。 Hybridizer Essentials 是免費的 Visual Studio 擴展 ,沒有硬件限制。 您可以在 GitHub 上找到一組基本的代碼示例和教育材料 。這些樣本也可以用來重現我們的性能結果。
在提供自動默認行為的同時, Hybridizer 在每個階段都提供了完全的開發人員控制,允許您重用現有的特定于設備的代碼、現有的外部庫或定制的手工代碼片段。
調試和分析
使用調試信息編譯時,可以在目標硬件上運行優化的代碼時,在 Microsoft Visual Studio 中調試 Hybridizer C #/. NET 代碼。例如,用 C 編寫的程序可以在 Visual Studio 中命中 C 文件中的斷點,并且可以探索駐留在 GPU 上的本地變量和對象數據。
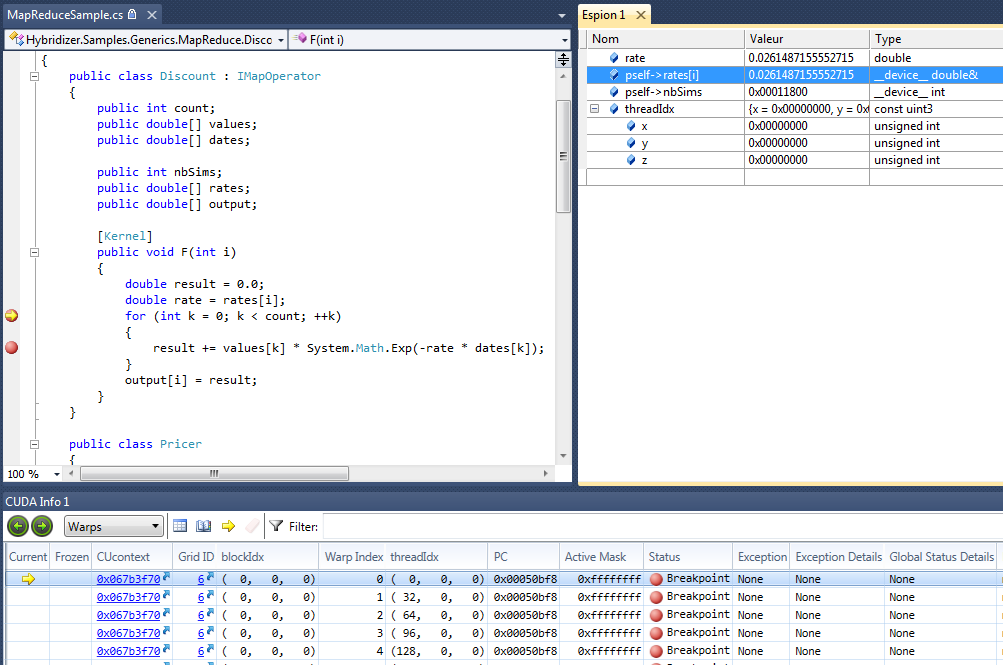
您可以在復雜的項目中集成 Hybridizer ,即使在代碼不可用或混淆的庫中也是如此,因為 Hybridizer 操作的是 MSIL 字節碼。我們在 ? 我們的博客帖子 中展示了這種能力,即在不修改庫的情況下,用雜交子加速大型圖像處理庫。在 MSIL 字節碼上操作還支持在. Net 虛擬機上構建的各種語言,例如 VB . Net 和 F 。
所有這些靈活性并不是以性能損失為代價的。正如我們的 ? benchmark 所說明的,雜交器生成的代碼可以執行與手寫代碼一樣好的性能。您可以使用性能分析器,如 NVIDIA Nsight 和 NVIDIA 可視化探查器來測量生成的二進制文件的性能,性能指標引用原始源代碼(例如 C )。
一個簡單的例子:曼德爾布洛特
作為第一個示例,我們演示了在 NVIDIA GeForce GTX 1080 Ti GPU ( Pascal 體系結構;計算能力 6 . 1 )上運行的 Mandelbrot 分形的渲染。
Mandelbrot C 代碼
下面的代碼片段顯示了純 C #。它在 CPU 上平穩運行,沒有任何性能損失,因為大多數代碼修改都是在運行時沒有影響的屬性(例如 Run
方法上的 EntryPoint
屬性)。
[EntryPoint] public static void Run(float[,] result) { int size = result.GetLength(0); Parallel2D.For(0, size, 0, size, (i, j) => { float x = fromX + i * h; float y = fromY + j * h; result[i, j] = IterCount(x, y); }); } public static float IterCount(float cx, float cy) { float result = 0.0F; float x = 0.0f, y = 0.0f, xx = 0.0f, yy = 0.0f; while (xx + yy <= 4.0f && result < maxiter) { xx = x * x; yy = y * y; float xtmp = xx - yy + cx; y = 2.0f * x * y + cy; x = xtmp; result++; } return result; }
EntryPoint
屬性告訴雜交器生成一個 CUDA 內核。多維數組映射到內部類型,而 Parallel2D.For
映射到 2D 執行網格。給出幾行樣板代碼,我們在 GPU 上透明地運行這段代碼。
float[,] result = new float[N,N]; HybRunner runner = HybRunner.Cuda("Mandelbrot_CUDA.dll").SetDistrib(32, 32, 16, 16, 1, 0); dynamic wrapper = runner.Wrap(new Program()); wrapper.Run(result);
描繪
我們使用 Nvidia Nsight Visual Studio Edition 探查器分析了這段代碼。 C 代碼在 CUDA 源代碼視圖中鏈接到 PTX ,如圖 3 所示。
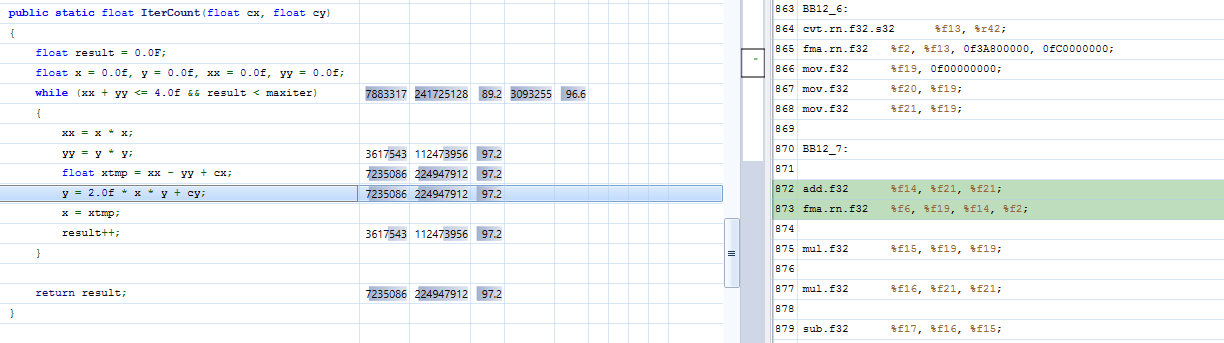
剖析器允許使用與 CUDA C ++代碼相同的調查級別。
至于性能,這個例子達到了峰值計算 FLOP / s 的 72 . 5% ,這是用 CUDA C++ 手寫的相同代碼的 83% 。
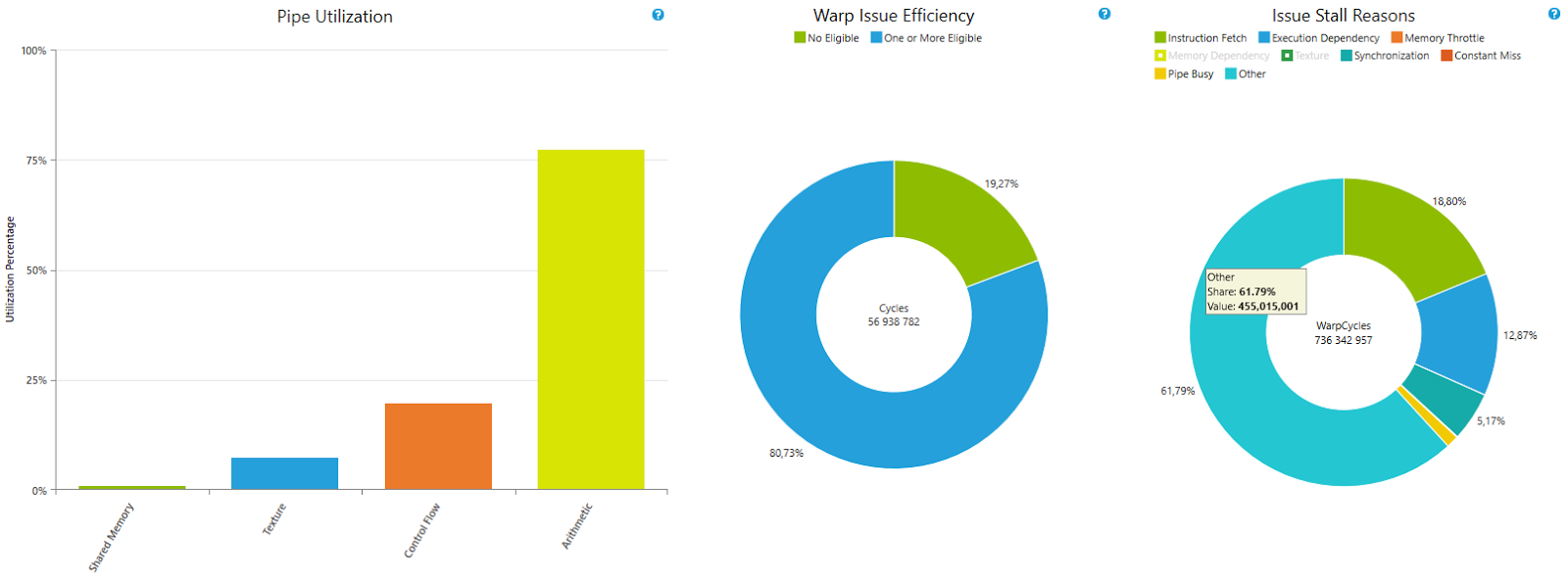
使用雜交器提供的擴展控制,可以從 C 代碼中獲得更好的性能。正如下面的代碼所示,語法與 CUDA C ++非常類似。
[EntryPoint] public static void Run(float[] result) { for (int i = threadIdx.y + blockIdx.y * blockDim.y; i < N; i += blockDim.y * gridDim.y) { for (int j = threadIdx.x + blockIdx.x * blockDim.x; j < N; j += blockDim.x * gridDim.x) { float x = fromX + i * h; float y = fromY + j * h; result[i * N + j] = IterCount(x, y); } } }
在這種情況下,生成的代碼和手寫的 CUDA C ++代碼執行一致,達到峰值觸發器的 87% ,如圖 5 所示。
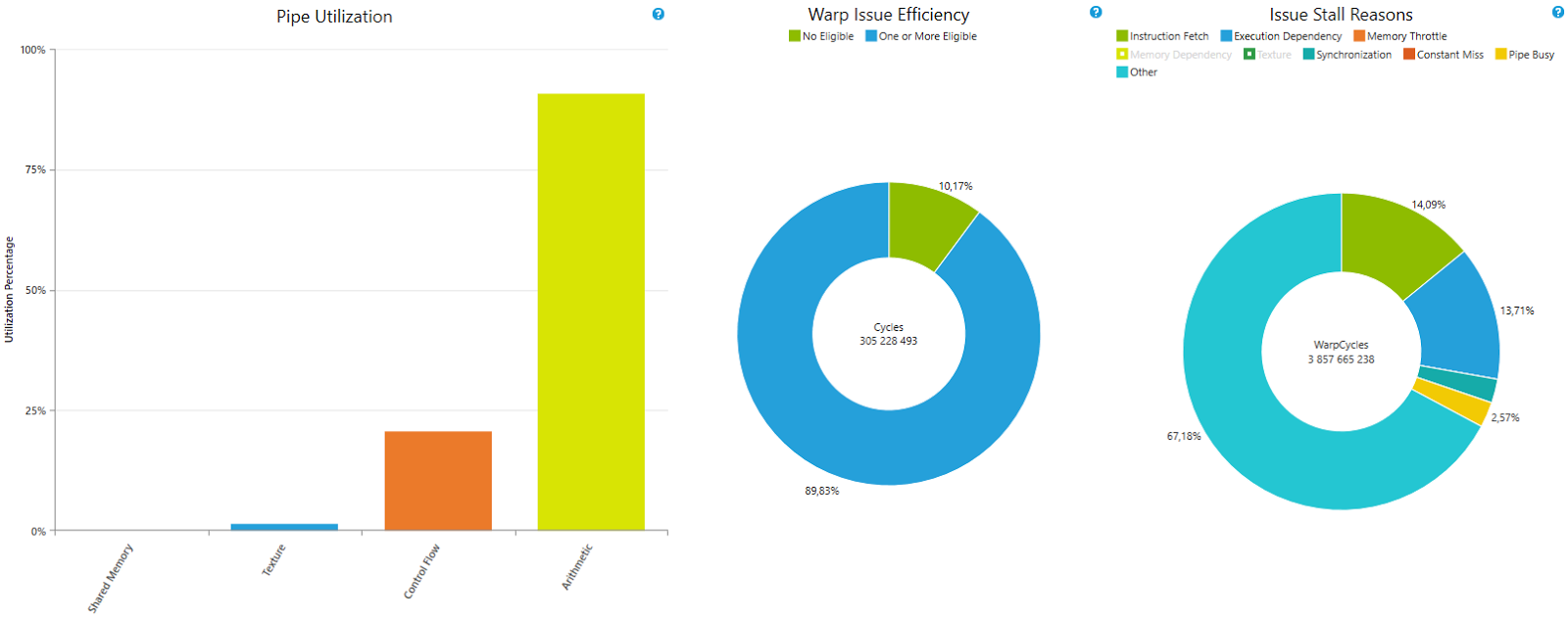
泛型與虛函數
Hybridizer 在設備功能上支持 泛型和虛函數調用 。現代編程語言的這些基本概念促進了代碼模塊化并提高了表達能力。然而, C 型的類型解析是在運行時完成的,這引入了一些性能上的懲罰。席。 NET- 泛型可以在保持靈活性的同時實現更高的性能: FixZER 將泛型映射到 C ++模板,這些模板在編譯時被解決,允許函數內聯和過程間優化。另一方面,虛擬函數中的方法被映射到另一個虛擬函數中。
模板實例化提示由兩個屬性 HybridTemplateConcept
和 HybridRegisterTemplate
提供給混合器(在設備代碼中觸發實際的模板實例化),另一個使用模板映射。基準依賴于一個公開下標運算符的公共接口 IMyArray
:
[HybridTemplateConcept] public interface IMyArray { double this[int index] { get; set; } }
這些操作員必須與設備功能“混合”。為此,我們將 Kernel
屬性放在實現類中。
public class MyArray : IMyArray { double[] _data; public MyArray(double[] data) { _data = data; } [Kernel] public double this[int index] { get { return _data[index]; } set { _data[index] = value; } } }
虛函數調用
在第一個版本中,我們使用接口編寫一個流算法,而不向編譯器提供進一步的提示。
public class MyAlgorithmDispatch { IMyArray a, b; public MyAlgorithmDispatch(IMyArray a, IMyArray b) { this.a = a; this.b = b; } [Kernel] public void Add(int n) { IMyArray a = this.a; IMyArray b = this.b; for (int k = threadIdx.x + blockDim.x * blockIdx.x; k < n; k += blockDim.x * gridDim.x) { a[k] += b[k]; } } }
因為我們在 a
和 b
上調用下標運算符,所以在 MSIL 中有一個 callvirt
。
IL_002a: ldloc.3 IL_002b: ldloc.s 4 IL_002d: callvirt instance float64 Mandelbrot.IMyArray::get_Item(int32) IL_0032: ldloc.1 IL_0033: ldloc.2 IL_0034: callvirt instance float64 Mandelbrot.IMyArray::get_Item(int32) IL_0039: add IL_003a: callvirt instance void Mandelbrot.IMyArray::set_Item(int32, float64)
檢查生成的二進制文件顯示, Hybridizer 在虛擬函數表中生成了一個查找,如圖 6 所示。
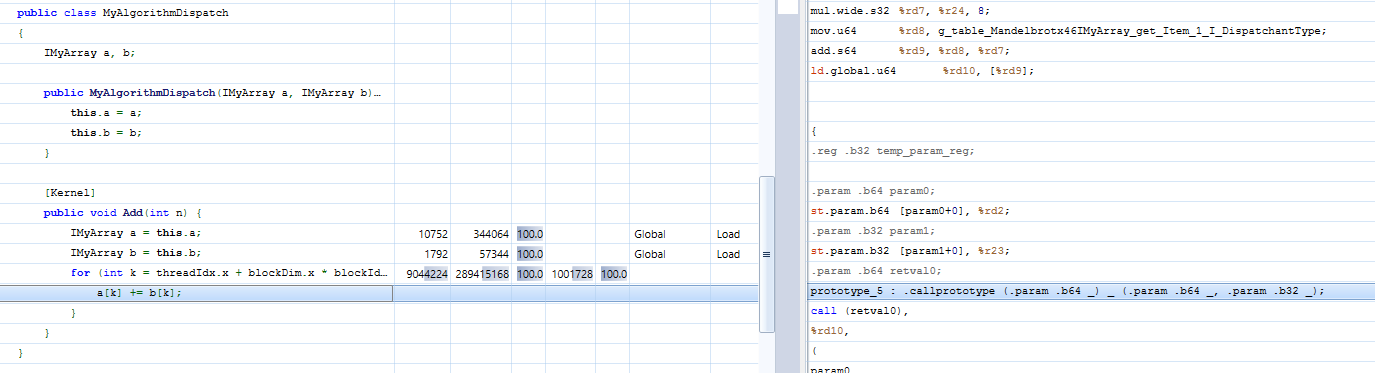
這個版本的算法消耗 32 個寄存器,達到 271 GB / s 的帶寬,如圖 7 所示。在相同的硬件上, CUDA 工具箱中的 bandwidthTest
示例達到 352Gb / s 。
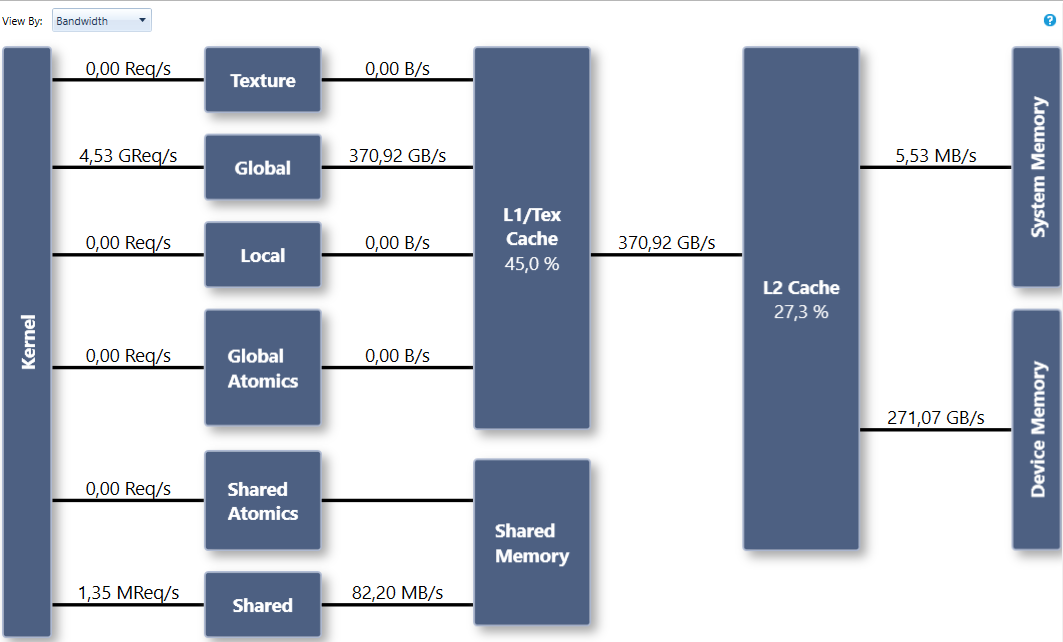
虛函數表導致更大的寄存器壓力,并阻止內聯。
一般呼叫
我們用泛型編寫了第二個版本,要求雜交子生成模板代碼。
[HybridRegisterTemplate(Specialize = typeof(MyAlgorithm))] public class MyAlgorithm where T : IMyArray { T a, b; [Kernel] public void Add(int n) { T a = this.a; T b = this.b; for (int k = threadIdx.x + blockDim.x * blockIdx.x; k < n; k += blockDim.x * gridDim.x) a[k] += b[k]; } } public MyAlgorithm(T a, T b) { this.a = a; this.b = b; } }
使用 RegisterTemplate
屬性, Hybridizer 生成適當的模板實例。然后優化器內聯函數調用,如圖 8 所示。
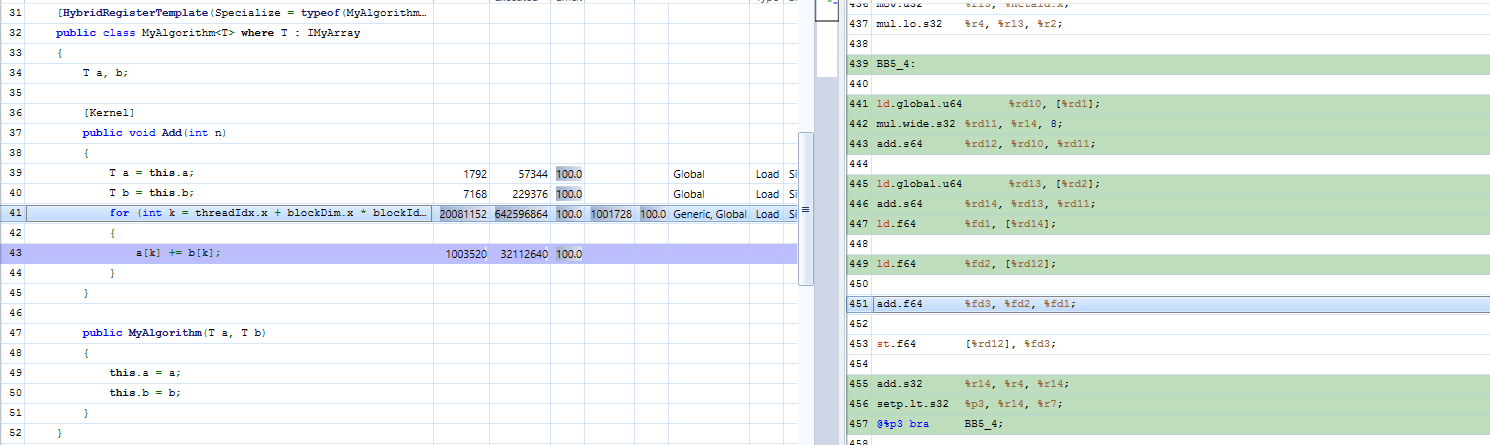
泛型參數的性能要好得多,達到 339gb / s ,性能提高了 25% (圖 9 ),比 bandwidthTest
提高了 96% 。
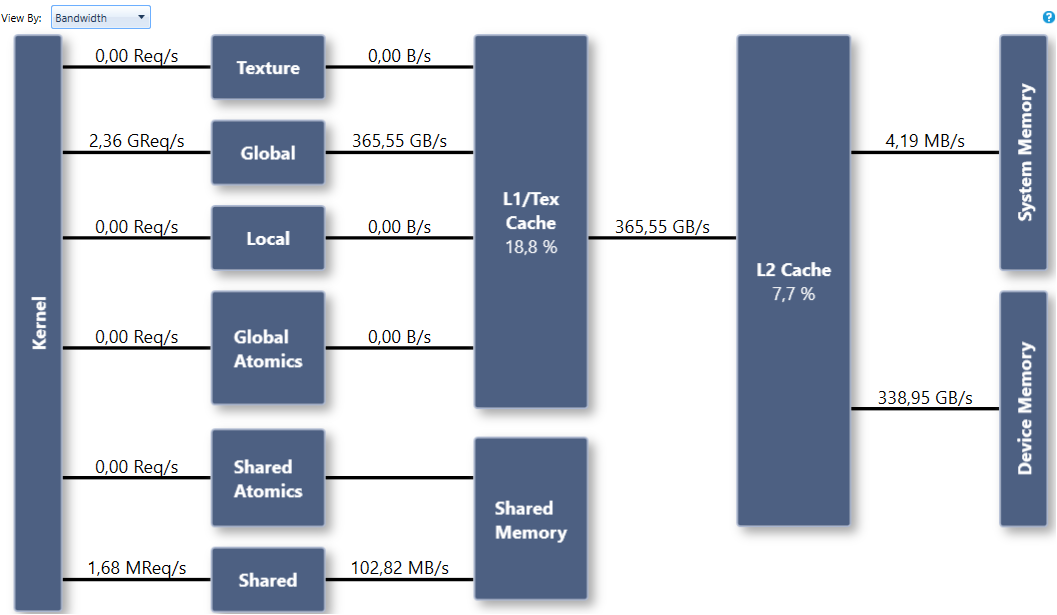
開始使用雜交劑
Hybridizer 支持多種 C 特性,允許代碼分解和表達能力。 Visual Studio 與 ? Nsight (調試器和探查器)的集成為您提供了一個安全高效的開發環境。 Hybridizer 即使在非常復雜、高度定制的代碼上也能獲得出色的 GPU 性能。
您可以從 Visual Studio 市場 下載 雜交者基本要素 。看看 github 上的 我們的 SDK 。
?