大多數 CUDA 開發人員都熟悉 cudaMalloc
和 cudaFree
API 函數來分配 GPU 可訪問內存。然而,這些 API 函數長期以來一直存在一個障礙:它們不是按流排序的。在本文中,我們將介紹新的 API 函數 cudaMallocAsync
和 cudaFreeAsync
,它們使內存分配和釋放成為流式有序操作。
在 本系列的第 2 部分 中,我們通過共享一些大數據基準測試結果來強調這一新功能的好處,并為修改現有應用程序提供代碼 MIG 定量指南。我們還介紹了在多 GPU 訪問和 IPC 使用環境中利用流順序內存分配的高級主題。這一切都有助于提高現有應用程序的性能。
流排序效率
下面左邊的代碼示例效率低下,因為第一個 cudaFree
調用必須等待 kernelA 完成,所以它會在釋放內存之前同步設備。為了提高運行效率,可以預先分配內存,并將其調整為兩種大小中的較大值,如右圖所示。
cudaMalloc(&ptrA, sizeA); kernelA<<<..., stream>>>(ptrA); cudaFree(ptrA); // Synchronizes the device before freeing memory cudaMalloc(&ptrB, sizeB); kernelB<<<..., stream>>>(ptrB); cudaFree(ptrB);
cudaMalloc(&ptr, max(sizeA, sizeB)); kernelA<<<..., stream>>>(ptr); kernelB<<<..., stream>>>(ptr); cudaFree(ptr);
這增加了應用程序中的代碼復雜性,因為內存管理代碼與業務邏輯分離。當涉及到其他圖書館時,問題就更加嚴重了。例如,考慮 kernelA 由庫函數啟動的情況,而不是:
libraryFuncA(stream); cudaMalloc(&ptrB, sizeB); kernelB<<<..., stream>>>(ptrB); cudaFree(ptrB); void libraryFuncA(cudaStream_t stream) { cudaMalloc(&ptrA, sizeA); kernelA<<<..., stream>>>(ptrA); cudaFree(ptrA); }
這對于應用程序來說要提高效率要困難得多,因為它可能無法完全查看或控制庫正在執行的操作。為了避免這個問題,庫必須在第一次調用該函數時分配內存,并且在庫被取消初始化之前永遠不會釋放內存。這不僅增加了代碼的復雜性,而且還會導致庫占用內存的時間超過需要的時間,從而可能會阻止應用程序的另一部分使用該內存。
有些應用程序通過實現自己的自定義分配器,進一步提前分配內存。這為應用程序開發增加了大量復雜性。 CUDA 旨在提供一種低工作量、高性能的替代方案。
CUDA 11 . 2 引入了流式有序內存分配器來解決這些類型的問題,并添加了 cudaMallocAsync
和 cudaFreeAsync
。這些新的 API 函數將內存分配從同步整個設備的全局作用域操作轉移到流順序操作,從而使您能夠將內存管理與 GPU 工作提交結合起來。這消除了同步未完成 GPU 工作的需要,并有助于將分配的生命周期限制為訪問它的 GPU 工作。考慮下面的代碼示例:
cudaMallocAsync(&ptrA, sizeA, stream); kernelA<<<..., stream>>>(ptrA); cudaFreeAsync(ptrA, stream); // No synchronization necessary cudaMallocAsync(&ptrB, sizeB, stream); // Can reuse the memory freed previously kernelB<<<..., stream>>>(ptrB); cudaFreeAsync(ptrB, stream);
現在可以在函數范圍內管理內存,如下面啟動 kernelA 的庫函數示例所示。
libraryFuncA(stream); cudaMallocAsync(&ptrB, sizeB, stream); // Can reuse the memory freed by the library call kernelB<<<..., stream>>>(ptrB); cudaFreeAsync(ptrB, stream); void libraryFuncA(cudaStream_t stream) { cudaMallocAsync(&ptrA, sizeA, stream); kernelA<<<..., stream>>>(ptrA); cudaFreeAsync(ptrA, stream); // No synchronization necessary }
流有序分配語義
所有常用的流排序規則都適用于 cudaMallocAsync
和 cudaFreeAsync
。從 cudaMallocAsync
返回的內存可以被任何內核或 memcpy
操作訪問,只要內核或 memcpy 被命令在分配操作之后和解除分配操作之前以流順序執行。解除分配可以在任何流中執行,只要命令在分配操作之后以及在 GPU 上對該內存的所有流進行所有訪問之后執行。
實際上,流順序分配的行為就像分配和自由是內核一樣。如果 kernelA 在流上生成有效緩沖區,并且 kernelB 在同一流上使其無效,則應用程序可以按照適當的流順序在 kernelA 之后和 kernelB 之前自由訪問緩沖區。
下面的示例顯示了各種有效用法。
auto err = cudaMallocAsync(&ptr, size, streamA); // If cudaMallocAsync completes successfully, ptr is guaranteed to be // a valid pointer to memory that can be accessed in stream order assert(err == cudaSuccess); // Work launched in the same stream can access the memory because // operations within a stream are serialized by definition kernel<<<..., streamA>>>(ptr); // Work launched in another stream can access the memory as long as // the appropriate dependencies are added cudaEventRecord(event, streamA); cudaStreamWaitEvent(streamB, event, 0); kernel<<<..., streamB>>>(ptr); // Synchronizing the stream at a point beyond the allocation operation // also enables any stream to access the memory cudaEventSynchronize(event); kernel<<<..., streamC>>>(ptr); // Deallocation requires joining all the accessing streams. Here, // streamD will be deallocating. // Adding an event dependency on streamB ensures that all accesses in // streamB will be done before the deallocation cudaEventRecord(event, streamB); cudaStreamWaitEvent(streamD, event, 0); // Synchronizing streamC also ensures that all its accesses are done before // the deallocation cudaStreamSynchronize(streamC); cudaFreeAsync(ptr, streamD);
圖 1 顯示了在前面的代碼示例中指定的各種依賴關系。如您所見,所有內核都被命令在分配操作之后執行,并在釋放操作之前完成。
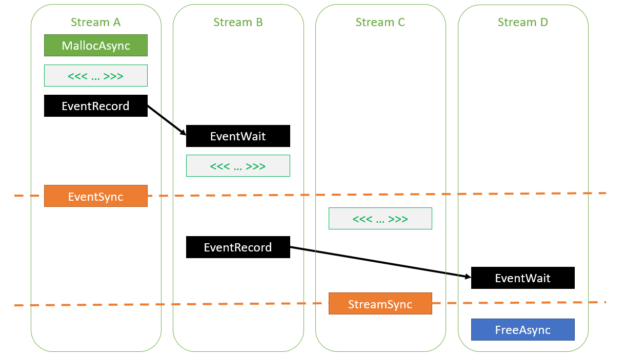
內存分配和釋放不能異步失敗。由于調用 cudaMallocAsync
或 cudaFreeAsync
(例如,內存不足)而發生的內存錯誤會通過調用返回的錯誤代碼立即報告。如果 cudaMallocAsync
成功完成,則返回的指針將保證是指向內存的有效指針,可以按照適當的流順序安全訪問。
err = cudaMallocAsync(&ptr, size, stream); if (err != cudaSuccess) { return err; } // Now you’re guaranteed that ‘ptr’ is valid when the kernel executes on stream kernel<<<..., stream>>>(ptr); cudaFreeAsync(ptr, stream);
CUDA 驅動程序使用內存池實現立即返回指針的行為。
內存池
流順序內存分配器將 存儲池 的概念引入 CUDA 。內存池是以前分配的內存的集合,可以重新用于將來的分配。在 CUDA 中,池由 cudaMemPool_t
句柄表示。每個設備都有一個默認池的概念,可以使用 cudaDeviceGetDefaultMemPool
查詢其句柄。
您還可以顯式創建自己的池,直接使用它們,或者將它們設置為設備的當前池,并間接使用它們。創建顯式池的原因包括自定義配置,如本文后面所述。當沒有顯式創建的池被設置為設備的當前池時,默認池將充當當前池。
在沒有顯式池參數的情況下調用 cudaMallocAsync
時,每次調用都會從指定的流推斷設備,并嘗試從該設備的當前池分配內存。如果池內存不足, CUDA 驅動程序將調用操作系統以分配更多內存。對 cudaFreeAsync
的每次調用都會將內存返回到池中,然后可在后續 cudaMallocAsync
請求中重新使用該內存。池由 CUDA 驅動程序管理,這意味著應用程序可以在多個庫之間實現池共享,而無需這些庫相互協調。
如果使用 cudaMallocAsync
發出的內存分配請求由于相應內存池的碎片而無法提供服務, CUDA 驅動程序通過將池中未使用的內存重新映射到 GPU 虛擬地址空間的連續部分來對池進行碎片整理。重新映射現有池內存而不是從操作系統分配新內存也有助于降低應用程序的內存占用。
默認情況下,在事件、流或設備上的下一次同步操作期間,池中累積的未使用內存將返回到操作系統,如下面的代碼示例所示。
cudaMallocAsync(ptr1, size1, stream); // Allocates new memory into the pool kernel<<<..., stream>>>(ptr); cudaFreeAsync(ptr1, stream); // Frees memory back to the pool cudaMallocAsync(ptr2, size2, stream); // Allocates existing memory from the pool kernel<<<..., stream>>>(ptr2); cudaFreeAsync(ptr2, stream); // Frees memory back to the pool cudaDeviceSynchronize(); // Frees unused memory accumulated in the pool back to the OS // Note: cudaStreamSynchronize(stream) achieves the same effect here
在池中保留內存
在某些情況下,將內存從池返回到系統可能會影響性能。考慮下面的代碼示例:
for (int i = 0; i < 100; i++) { cudaMallocAsync(&ptr, size, stream); kernel<<<..., stream>>>(ptr); cudaFreeAsync(ptr, stream); cudaStreamSynchronize(stream); }
默認情況下,流同步會導致與該流的設備關聯的任何池將所有未使用的內存釋放回系統。在本例中,這將在每次迭代結束時發生。因此,沒有內存可供下次 cudaMallocAsync
調用重用,而必須通過昂貴的系統調用來分配內存。
為了避免這種昂貴的重新分配,應用程序可以配置一個釋放閾值,以使未使用的內存在同步操作之后保持不變。釋放閾值指定池緩存的最大內存量。在同步操作期間,它會將所有多余的內存釋放回操作系統。
默認情況下,池的釋放閾值為零。這意味著池中使用的內存在每次同步操作期間都會釋放回操作系統。下面的代碼示例演示如何更改釋放閾值。
cudaMemPool_t mempool; cudaDeviceGetDefaultMemPool(&mempool, device); uint64_t threshold = UINT64_MAX; cudaMemPoolSetAttribute(mempool, cudaMemPoolAttrReleaseThreshold, &threshold); for (int i = 0; i < 100; i++) { cudaMallocAsync(&ptr, size, stream); kernel<<<..., stream>>>(ptr); cudaFreeAsync(ptr, stream); cudaStreamSynchronize(stream); // Only releases memory down to “threshold” bytes }
使用非零釋放閾值可以從一個迭代到下一個迭代重用內存。這只需要簡單的簿記,并使 cudaMallocAsync
的性能獨立于分配的大小,從而顯著提高了內存分配性能(圖 2 )。
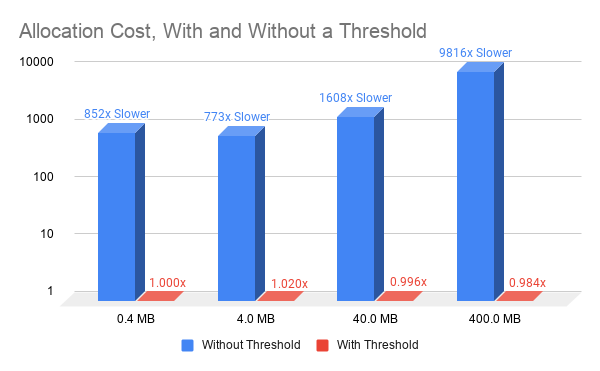
池閾值只是一個提示。在相同的內存池中[0]可以隱式釋放內存分配,以使內存分配成功。例如,對 cudaMalloc
或 cuMemCreate
的調用可能會導致 CUDA 從與同一進程中的設備關聯的任何內存池中釋放未使用的內存來為請求提供服務
這在應用程序使用多個庫的情況下尤其有用,其中一些庫使用 cudaMallocAsync
,而另一些庫不使用 cudaMallocAsync
。通過自動釋放未使用的池內存,這些庫不必相互協調以使各自的分配請求成功。
CUDA 驅動程序自動將內存從池重新分配給不相關的分配請求時存在限制。例如,應用程序可能使用不同的接口(如 Vulkan 或 DirectX )來訪問 GPU ,或者可能有多個進程同時使用 GPU 。這些上下文中的內存分配請求不會自動釋放未使用的池內存。在這種情況下,應用程序可能必須通過調用 cudaMemPoolTrimTo
顯式釋放池中未使用的內存。
size_t bytesToKeep = 0; cudaMemPoolTrimTo(mempool, bytesToKeep);
bytesToKeep
參數告訴 CUDA 驅動程序它可以在池中保留多少字節。任何超過該大小的未使用內存都會釋放回操作系統。
通過內存重用提高性能
cudaMallocAsync
和 cudaFreeAsync
的 stream 參數有助于 CUDA 高效地重用內存,避免對操作系統進行昂貴的調用。考慮下面的瑣碎代碼示例。
cudaMallocAsync(&ptr1, size1, stream); kernelA<<<..., stream>>>(ptr1); cudaFreeAsync(ptr1, stream); cudaMallocAsync(&ptr2, size2, stream); kernelB<<<..., stream>>>(ptr2);
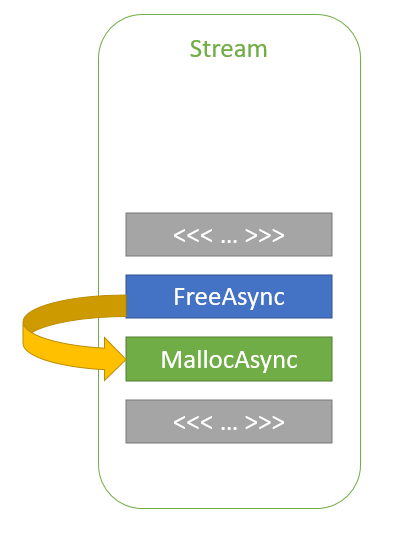
在這個代碼示例中, ptr2
是在 ptr1
被釋放后按流順序分配的。 ptr2
分配可以重用用于 ptr1
的部分或全部內存,而無需任何同步,因為 kernelA 和 kernelB 在同一個流中啟動。因此,流排序語義保證 kernelB 在 kernelA 完成之前不能開始執行和訪問內存。通過這種方式, CUDA 驅動程序可以幫助降低應用程序的內存占用,同時提高分配性能。
CUDA 驅動程序還可以跟蹤通過 CUDA 事件插入的流之間的依賴關系,如以下代碼示例所示:
cudaMallocAsync(&ptr1, size1, streamA); kernelA<<<..., streamA>>>(ptr1); cudaFreeAsync(ptr1, streamA); cudaEventRecord(event, streamA); cudaStreamWaitEvent(streamB, event, 0); cudaMallocAsync(&ptr2, size2, streamB); kernelB<<<..., streamB>>>(ptr2);
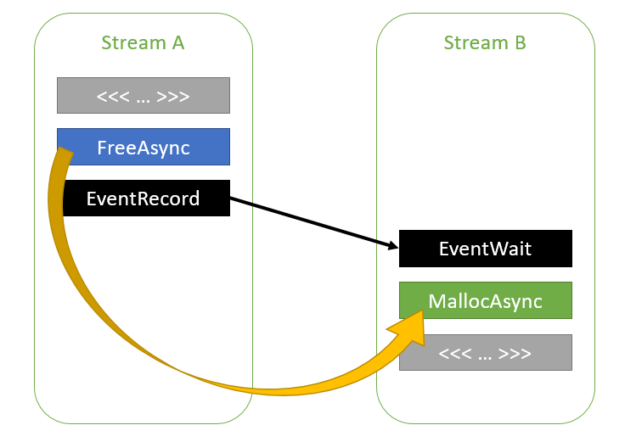
由于 CUDA 驅動程序知道流 A 和 B 之間的依賴關系,因此它可以重用 ptr1
為 ptr2
使用的內存。流 A 和 B 之間的依賴關系鏈可以包含任意數量的流,如下面的代碼示例所示。
cudaMallocAsync(&ptr1, size1, streamA); kernelA<<<..., streamA>>>(ptr1); cudaFreeAsync(ptr1, streamA); cudaEventRecord(event, streamA); for (int i = 0; i < 100; i++) { cudaStreamWaitEvent(streams[i], event, 0); // streams[] is a previously created array of streams cudaEventRecord(event, streams[i]); } cudaStreamWaitEvent(streamB, event, 0); cudaMallocAsync(&ptr2, size2, streamB); kernelB<<<..., streamB>>>(ptr2);
如有必要,應用程序可以基于每個池禁用此功能:
int enable = 0; cudaMemPoolSetAttribute(mempool, cudaMemPoolReuseFollowEventDependencies, &enable);
CUDA 驅動程序還可以在沒有應用程序指定的顯式依賴項的情況下,有機會重用內存。雖然這種啟發式方法可能有助于提高性能或避免內存分配失敗,但它們會給應用程序增加不確定性,因此可以在每個池的基礎上禁用。考慮下面的代碼示例:
cudaMallocAsync(&ptr1, size1, streamA); kernelA<<<..., streamA>>>(ptr1); cudaFreeAsync(ptr1); cudaMallocAsync(&ptr2, size2, streamB); kernelB<<<..., streamB>>>(ptr2); cudaFreeAsync(ptr2);
在此場景中, streamA 和 streamB 之間沒有明確的依賴關系。但是, CUDA 驅動程序知道每個流執行了多遠。如果在第二次調用 streamB 中的 cudaMallocAsync
時, CUDA 驅動程序確定 kernelA 已在 GPU 上完成執行,則它可以重用 ptr1
用于 ptr2
的部分或全部內存。
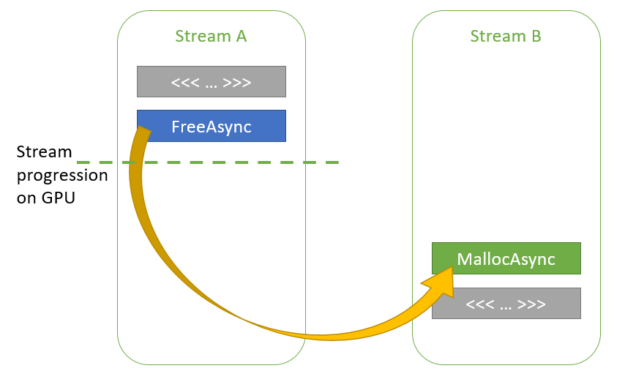
如果 kernelA 尚未完成執行, CUDA 驅動程序可以在兩個流之間添加隱式依賴項,以便 kernelB 在 kernelA 完成之前不會開始執行。
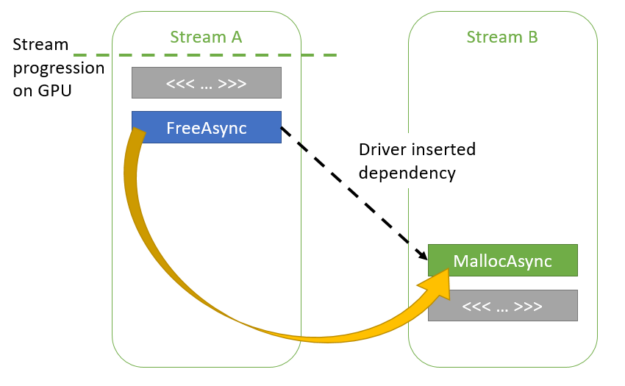
應用程序可以按如下方式禁用這些啟發式:
int enable = 0; cudaMemPoolSetAttribute(mempool, cudaMemPoolReuseAllowOpportunistic, &enable); cudaMemPoolSetAttribute(mempool, cudaMemPoolReuseAllowInternalDependencies, &enable);
概括
在本系列的第 1 部分中,我們介紹了新的 API 函數 cudaMallocAsync
和 cudaFreeAsync
,這兩個函數使內存分配和釋放成為流順序操作。使用它們可以避免通過 CUDA 驅動程序維護的內存池對操作系統進行昂貴的調用。
在 本系列的第 2 部分 中,我們分享了一些基準測試結果,以展示流順序內存分配的好處。我們還提供了一個逐步修改現有應用程序的方法,以充分利用此高級 CUDA 功能。
?